
Here, the DOAs are calculated with eigenrays from a ray model. Practically, the DOAs are estimated with various beamforming schemes. In this work, beamforming results from the array are used with CS to infer the SSP.

It inverts the SSP with enhanced signal-to-noise ratio (SNR) due to the beamforming and without intensive use of propagation model as well as geoacoustic parameters. This work suggests a novel inversion scheme for SSP, which employs compressive sensing (CS) with beamforming results (i.e., direction of arrivals (DOAs)).
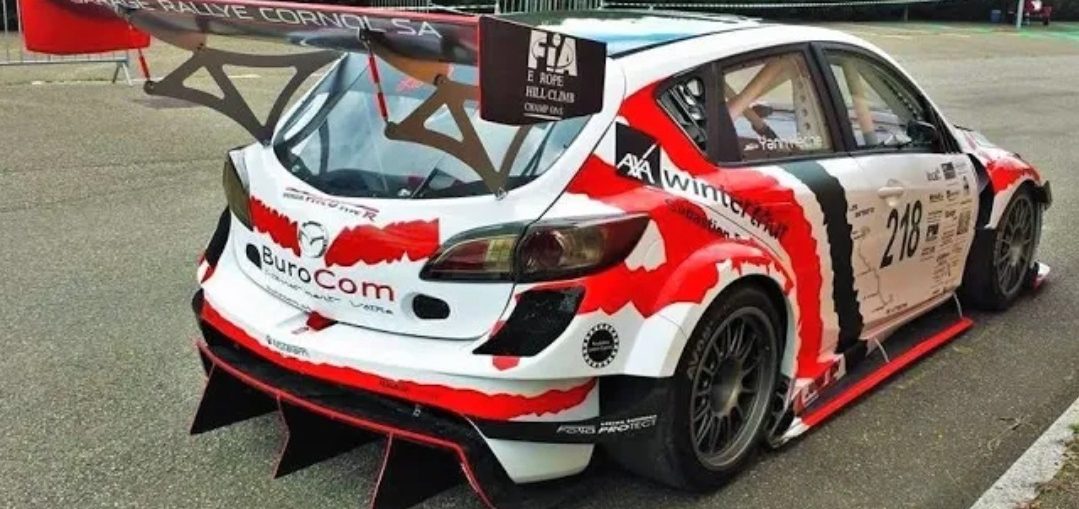
However, its performance relies on the propagation model and it cannot be applied to environments, where the propagation model is restricted owing to complexities of the ocean including geoacoustic parameters (sound speed, density, and attenuation) of sediment. It estimates the SSP more robustly at the cost of computational burden resulting from iterative use of propagation model. In general, a genetic algorithm or simulated annealing is applied to solve the optimization problem. The replicas corresponding to the maximum (or minimum) value from the objective function should then resemble the SSP from the ocean. In the SSP inversion process, replicas (synthetic acoustic data at the sensors of array) are generated according to the SSPs using underwater acoustic propagation model, and an objective function is defined to evaluate the similarity between the measured data and the replicas. To mitigate the problem, multiple measurement at sensors in array is used with matched field inversion (MFI). Its application is limited by ocean noises that mask the arrivals. Originally, ocean acoustic tomography is conducted using travel times of arrivals measured at a single hydrophone. Many studies have used acoustic measurement data to infer the SSP (i.e., acoustic tomography). It is crucial to estimate the sound speed profile (SSP) of a water column to reliably locate a source or to conduct geoacoustic inversion. Five hundred synthetic SSPs were generated by randomly choosing the SSP dictionary components, and more than 80 percent of all the cases have correlation coefficients over 0.7 and MSE along depth is insignificant except near the sea surface, which shows the validity of the proposed method. The SSP is estimated using CS with beamforming of ray arrivals in the SWellEx-96 experimental environment, and the performance is evaluated using the correlation coefficient and mean squared error (MSE) between the true and recovered SSPs, respectively. The conventional objective function in CS is modified to simultaneously exploit the information from the travel times and positions.
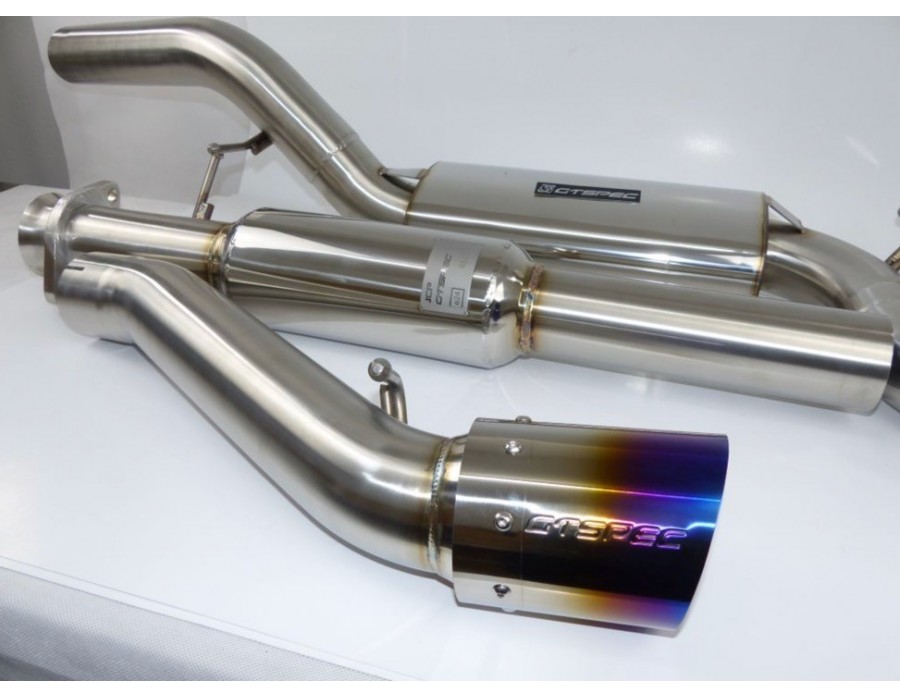
The linear relation between the travel times/positions and the shape function coefficients enables CS to reconstruct the SSP. The travel times and the positions of the arrivals can be approximately linearized using their Taylor expansion with the shape function coefficients that parameterize the SSP. In this work, the SSP is inverted using compressive sensing (CS) combined with beamforming to indicate the direction of arrivals (DOAs). Sound speed profile (SSP) significantly affects acoustic propagation in the ocean.
